DC-ren
DC-ren
Overview
Personalization & Precision Medicine in chronic diseases
Current medical practice relies on a catalogue of diseases, each defined by pathophysiology, symptoms and outcomes. In case of strict causality, a specific diagnosis has one clinical consequence and this “action – reaction” scheme then applies for all subjects affected. Individual variance in disease progression and response to treatment is considered limited. For many and especially chronic and age-associated diseases however the situation is more intricate. While the sequence of symptoms, pathophysiology, treatment and outcome still holds true for a cohort, we observe clinically inter-individual and longitudinal intra-individual heterogeneity in disease progression as well as response to therapy. In this scenario, precision in diagnosis and treatment needs improvement by fostering repeated stratification and personalization, particularly if different treatment options are available.
The latter is the aim of the collaborative R&D initiative DC-ren in an extremely relevant disorder: Diabetic Kidney Disease (DKD). DC-ren, the abbreviation for “Drug combinations for rewriting trajectories of renal pathologies in type II diabetes”, has started in 2020 with a runtime of 5 years. DC-ren has received funding from the European Union’s Horizon 2020 research and innovation programme under grant agreement No 848011.
Diabetic Kidney Disease
DC-ren focuses on DKD, a severe long–term complication of type II diabetes mellitus (T2DM), which is characterized by alterations in glucose metabolism caused by insulin resistance. DKD, a gradual loss of renal function leading to end stage renal failure, is per se a complex disorder, which is further modulated by a high burden of co-morbidities. Complexity is also evident on the level of pathophysiology and guidelines recommend drug combination therapy. In recent years, treatment choices have increased, with several novel drugs with proven renal as well as cardiovascular benefit entering the market. Patients with DKD display remarkable inter-, and longitudinal intra-individual variability in disease progression and response to certain drug combinations. Thus, there is an evident clinical need for adding precision in treatment via personalization. The central objective of DC-ren is to provide a decision support technology for selecting the optimal drug combination treatment to improve the prognosis on the level of individual patients with DKD.
While pursuing a clear focus on DKD, we are confident that our novel methodological approaches have the potential for serving as blueprint for adding precision in treatment of complex chronic disorders in general.
”The good physician treats the disease; the great physician treats the patient who has the disease.
Sir William Osler, 1903
DC-ren
Concept
Disease & treatment: a dynamical system
Guidelines recommend regular monitoring of renal excretory function in T2DM by a laboratory parameter called “eGFR”. Of note, not all patients with T2DM develop DKD, and those who do loose eGFR with a remarkable inter-, but also intra-individual variability over many years. This is due to the complex interaction of genetic predisposition and environmental factors resulting in a personalized trajectory of development and progression of DKD.
Formally, DKD evolves as a sequence of pathophysiological states being causally linked by action – reaction. Certain trajectories resemble a fast decline of eGFR while others result in a fairly stable situation. Inter-individual heterogeneity is further complicated by intra-individual variability. Some states may result in stable or even improving renal function, others in rapid decline and some may see rapid transition while others are stable for years. A state is defined by its underlying pathophysiology, and we have to keep in mind that this is the point of action of drugs. Therefore, drugs exhibit beneficial effects only in certain states, while they fail in others. When applying such a state model we can capture inter-, but also intra-individual variance in drug response.
DC-ren follows the concept of individualized trajectories of state transitions with state-specific drug response according to underlying pathophysiology. Such a setting is conceptually well established in the scientific domain of dynamical systems research.
Implications
According to a dynamical systems approach (individualized trajectories of state evolution combined with state-specific drug response) the DC-ren work plan takes care of three central R&D aspects:
Goals
Application goals
The translational focus of DC-ren is to establish and validate a decision support software application for optimizing combination drug therapy in patients with DKD on a personalized level in a “technology readiness level 6” prototype (i.e. allowing practical demonstration in a relevant environment).
For implementation a validation study, leveraging on existing clinical trial repositories and realized as a “virtual trial”, will test if technology-mediated decisions add in precision in personalized drug response prediction when compared to present clinical guidelines. This validation is pivotal for moving the decision support solution in higher technology readiness levels, and ultimately in clinical settings.
On top, we test the decision support solution in two relevant application scenarios, namely in DKD drug R&D and compound recovery:
Science goals
All science in DC-ren is geared towards implementing the technology solution. The toolbox elements come from analysis of a high-dimensional data space covering clinical and molecular characteristics for a substantial cohort of DKD patients. Here we need to capture details of the personalized disease pathophysiology at the interface of mechanism of action of drug combinations on a common layer of molecular processes, which interact as a System-of-Systems. The challenge is to identify molecular biomarkers and clinical parameters being informative on drug effect in dependence of underlying process configuration for state-specific pathophysiologies. For coming up with an ideal biomarker panel we combine in-silico based candidate biomarker selection with an explorative proteomics approach. The data space shall serve for generalizing and inferring the consequence of the drug effect on personalized (state-specific) pathophysiology, ultimately allowing statements on optimal drug combination matches.
Statements on the level of the toolbox are derived from a hybrid AI. “Hybrid” refers to a combination of data-driven generalization from statistics, machine learning and rule-based inference from simulation. Such an approach shall allow us to streamline probabilistic predictions with causal (action – reaction) statements on drug response. “Data rich” analysis up to learning has become highly acclaimed in recent years also for solving medical challenges. We believe this methods compendium needs expansion with rule-based, mechanistic approaches, as causality and determinism are pivotal for bringing decisions on optimal drug combinations forward in precision medicine.
DC-ren
Team
Interdisciplinary R&D
Contemporary clinical research critically depends on interdisciplinary teams, and DC-ren balances theoretical, experimental and clinical expertise. The DC-ren analytical concept routes in applied Category Theory, a framework providing concepts and tools for modeling dynamical systems. Its specific strength is the representation of composite function, in DC-ren being distinct DKD pathologies at interference with drug mechanism of action. Translating the concept via combining statistics and simulation on the basis of a rich clinical and molecular data space allows taking a novel perspective for assessing personalized drug response as hybrid AI solution. We leverage on extensive biobanks and clinical data repositories readily capturing personalized response to various drugs approved for DKD.
We complement clinical phenotyping with top-level molecular profiling, going for multiplexed assays and proteomics. This will provide us with a targeted data matrix for capturing disease mechanisms and drug impact at the effector level of molecular processes: A System-of-Systems. All analytical and experimental work is embedded in strong clinical research for assuring technology development right in focus of patient needs. Practical implementation follows agile software development tailored at reaching a prototype decision support platform, finally evaluated in dedicated validation and DKD drug application studies. With this setting DC-ren bridges from a novel concept to a clinical solution with substantial technology readiness level.
Partner institutions
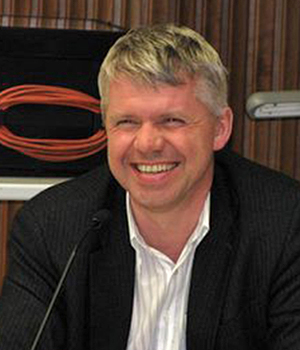
PI: Gert Mayer
DC-ren biomarker quantitation by targeted proteomics and multiplexed assays.
The central objective of DC-ren is to provide a decision support tool for selecting the optimal drug combination treatment to improve the prognosis on the level of individual patients with diabetes mellitus type 2 and DKD. The technology rests on biomarkers selected by combining bioinformatical analysis of DKD pathophysiology and drug mode of action. DC-ren project partners recently successfully executed the biomarker panel quantitation by applying state of the art technologies.
Execution of targeted proteomics.
Urinary proteomics analysis was performed at Mosaiques diagnostics, Hannover, Germany, headed by Harald Mischak, a leading authority in clinical proteomics and biomarker identification. Mosaiques revolutionary CE-MS technology (capillary electrophoresis coupled to mass spectrometry) assessed more than 21.000 urinary peptides. Mosaiques diagnostics provides highly innovative Clinical Proteomics services for academic institutions and pharmaceutical companies.
Execution of multiplexed assays.
A DC-ren specific targeted protein biomarker panel in the context of drug response and DKD progression was established for quantitation in blood and urine samples of DKD patients with diabetes mellitus type 2.
The research group of the Nephrology Laboratory of Rainer Oberbauer at the Department of Medicine III, Medical University Vienna, Austria, performed the Luminex assays for biomarker measurements in serum and plasma.
The Mesoscale platform was utilized for urinary biomarker measurements and performed by the scientific DKD team of the Laboratory of the Department of Internal Medicine IV (Nephrology and Hypertension), Medical University Innsbruck, Austria headed by Gert Mayer.
Partner institutions
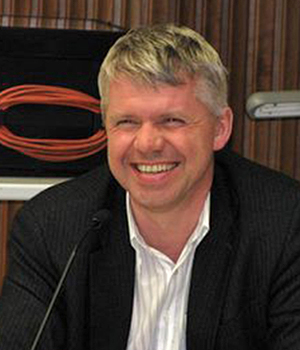
PI: Gert Mayer
Recent News
Publications
DC-ren publications
Failure of biomarkers to personalize clinical practice in diabetic kidney disease. Are we asking the right questions?
Denicolò S, Keller F, Thöni S, Mayer G, Mayer B. Nehro-News 02/2020; 17-21.
Precision medicine in transplantation and hemodialysis.
Oberbauer R, Meyer TW. Nephrol Dial Transplant. 2021 Jun 22;36(Suppl 2):31-36.
Precision medicine approaches for diabetic kidney disease: opportunities and challenges.
Sok Cin T, Denig P, Heerspink HJL. Nephrol Dial Transplant. 2021 Jun 22;36(Suppl 2):3-9.
Non-adherence to antidiabetic and cardiovascular drugs in type 2 diabetes mellitus and its association with renal and cardiovascular outcomes: A narrative review.
Denicolo S, Thöni S, Perco P, Mayer G. J Diabetes Complications. 2021 Jul;35(7):10793.
Clustering longitudinal data with category theory for diabetic kidney disease.
Mannone M, Distefano V, Silvestri C, Poli I. CLADAG 2021: Book of abstracts and short papers.
Clustering longitudinal data on diabetic kidney disease.
Mannone M, Distefano V, Silvestri C, Poli I. In CLADAG, eds. Porzio et al., Firenze University Press 2021 (ISSN 2704-601X – ISSN).
Categories and clusters to investigate similarities in diabetic kidney disease patients.
Distefano V, Mannone M, Silvestri C, Poli I. SIS 2021: Book of short papers.
Editorial: precision medicine in nephrology.
Mayer G. Nephrol Dial Transplant. 2021 Jun 22;36(Suppl 2):1-2. doi: 10.1093/ndt/gfaa366.
Clonal hematopoiesis of indeterminate potential and diabetic kidney disease: a nested case-control study.
Denicolò S, Vogi V, Keller F, Thöni S, Eder S, Heerspink HL, Rosivall L, Wiecek A, Mark PB, Perco P, Leierer J, Kronbichler A, Steger M, Schwendinger S, Zschocke J, Mayer G, Jukic E. Kidney Int Rep. 2022 Feb 3;7(4):876-888. doi: 10.1016/j.ekir.2022.01.1064. eCollection 2022 Apr.
Biological variation and reference change value of the estimated glomerular filtration rate in humans: A systematic review and meta-analysis.
Thöni S, Felix K, Denicolò S, Buchwinkler L, Mayer G. Front Med (Lausanne). 2022 Oct 6;9:1009358. doi: 10.3389/fmed.2022.1009358. eCollection 2022.
Assessment of Fibrinogen-like 2 (FGL2) in Human Chronic Kidney Disease through Transcriptomics Data Analysis.
Denicolò S, Nair V, Leierer J, Rudnicki M, Kretzler M, Mayer G, Ju W, Perco P. Biomolecules. 2022 Dec 31;13(1):89. doi: 10.3390/biom13010089.
Presence of retinopathy and incident kidney and cardiovascular events in type 2 diabetes with normoalbuminuria – A post-hoc analysis of the PRIORITY randomized clinical trial.
Curovic VR, Tofte N, Lindhardt M, Adamova K, Bakker SJL, Beige J, Beulens JWJ, Birkenfeld AL, Currie Gl, Delles C, Dimos I, Francová L, Frimodt-Møller M, Girman P, Göke R, Hansen TW, Havrdova T, Kooy A; Laverman GD, Mischak H, Navis G, Nijpels G, Noutsou M, Ortiz A, Parvanova A, Persson F, Petrie JR, Ruggenenti PL, Rutters F, Rychlík I, Siwy J, Spasovski G, Speeckaert M, Trillini M, Zürbig P, von der Leyen H, Rossing P, on the behalf of the PRIORITY Study Group. J Diabetes Complications. 2023 Apr;37(4):108433. doi: 10.1016/j.jdiacomp.2023.108433. Epub 2023 Feb 18.
International Variability of Renal and Cardiovascular Outcomes and Mortality in Patients with Type 2 Diabetes Mellitus in Europe.
Exploring Heterogeneity with Category and Cluster Analyses for Mixed Data.
Effect of treatment with GLP-1R agonists on the urinary peptidome of T2DM patients.
Lohia S, Siwy J, Mavrogeorgis E, Eder S, Thoeni S, Mayer G, Mischak H, Vlahou A, Jankowski V. Authorea. June 06, 2023. doi: 10.22541/au.168607087.71676303/v1.
Candidate composite biomarker to inform drug treatments for diabetic kidney disease.
Jones RD, Abebe S, Distefano V, Mayer G, Poli I, Silvestri C, Slanzi D. Front Med (Lausanne). 2023 Nov 1; 10: 1271407. doi: 10.3389/fmed.2023.1271407.
Exploratory Study Analyzing the Urinary Peptidome of T2DM Patients Suggests Changes in ECM but Also Inflammatory and Metabolic Pathways Following GLP-1R Agonist Treatment.
Lohia S, Siwy J, Mavrogeorgis E, Eder S, Thöni S, Mayer G, Mischak H, Vlahou A, Jankowski V. Int J Mol Sci. 2023 Aug 31;24(17):13540. doi: 10.3390/ijms241713540.
Prognosis and Personalized In Silico Prediction of Treatment Efficacy in Cardiovascular and Chronic Kidney Disease: A Proof-of-Concept Study.
Campos MAJ, Andújar I, Keller F, Mayer G, Rossing P, Staessen JA, Delles C, Beige J, Glorieux G, Clark AL, Mullen W, Schanstra JP, Vlahou A, Rossing K, Peter K, Ortiz A, Campbell A, Persson F, Latosinska A, Mischak H, Siwy J, Jankowski J. Pharmaceuticals (Basel). 2023 Sep 14;16(9):1298. doi: 10.3390/ph16091298.
Randomized Trial of SGLT2 Inhibitor Identifies Target Proteins in Diabetic Kidney Disease.
Ahluwalia TS, Rönkkö TKE, Eickhoff MK, Curovic VR, Siwy J, Eder S, Denicolò S, Mayer G, Mischak H, Rossing P, Persson F. Kidney Int Rep. 2024 Feb 2;9(2):334-346 doi:10.1016/j.ekir.2023.11.020
Clustering Trajectories to Study Diabetic Kidney Disease.
Distefano V, Mannone M, Poli I, Mayer G. In: Villani, M., Cagnoni, S., Serra, R. (eds) Artificial Life and Evolutionary Computation. WIVACE 2023. Communications in Computer and Information Science, vol 1977. Springer, Cham. Conference Paper, March 2024. doi: 10.1007/978-3-031-57430-6_21.
Exploiting the Potential of Bayesian Networks in Deriving New Insight into Diabetic Kidney Disease (DKD).
Slanzi D, Silvestri C, Poli, I, Mayer G. In: Villani, M., Cagnoni, S., Serra, R. (eds) Artificial Life and Evolutionary Computation. WIVACE 2023. Communications in Computer and Information Science, vol 1977. Springer, Cham. Conference Paper, March 2024. doi: 10.1007/978-3-031-57430-6_23.
Urinary peptide analysis to predict the response to blood pressure medication.
Campos MAJ, Mavrogeorgis E, Latosinska A, Eder S, Buchwinkler L, Mischak H, Siwy J, Rossing P, Mayer G, Jankowski J..Nephrol Dial Transplant. 2024 Apr 26;39(5):873-883. doi: 10.1093/ndt/gfad223.
Non-adherence to cardiometabolic medication as assessed by LC-MS/MS in urine and its association with kidney and cardiovascular outcomes in type 2 diabetes mellitus.
Denicolò S, Reinstadler V, Keller F, Thöni S, Eder S, Heerspink HJL, Rosivall L, Wiecek A, Mark PB, Perco P, Leierer J, Kronbichler A, Oberacher H, Mayer G. Diabetologia. 2024 Jul;67(7):1283-1294. doi: 10.1007/s00125-024-06149-w.
Learning Optimal Dynamic Treatment Regime From Observational Clinical Data Through Reinforcement Learning.
Abebe S, Poli I , Jones RD and Slanzi D. Mach. In: Learn. Knowl. Extr. 2024, July 25:1, 1–20.
Association of urinary EGF, FABP3, and VCAM1 levels with the progression of early diabetic kidney disease.
Keller F, Denicolò S, Leierer J, Kruus M, Heinzel A, Kammer M, Ju W, Nair V, Burdet F, Ibberson M, Menon R, Otto E, Choi YJ, Pyle L, Ladd P, Bjornstad PM, Eder S, Rosivall L, Mark PB, Wiecek A, Heerspink HJL, Kretzler M, Oberbauer R, Mayer G, Perco P. Kidney Blood Press Res. 2024 Nov 7:1-21.
DC-ren associated studies
The concept of precision medicine as proposed by DC-ren is also of great interest to SMEs and other academic institutions. In order to allow collaborations, we established the concept of “DC-ren associated studies”.
Currently DC-ren collaborates with the Department of Nephrology, Saarland University Medical Center, Homburg, Germany to evaluate the short and long term prognostic and predictive power of urinary Dickkopf-3 in patients with type 2 diabetes mellitus.
In addition the DC-ren team was approached by Nordic Bioscience® (based in Herlev, Denmark), a company focusing amongst others on biomarkers of extracellular matrix turnover, a pathomechanism of great interest in the context of drug response and DKD progression. The DC-ren consortium and Nordic Bioscience agreed to collaborate on projects looking at the association of novel extracellular matrix turnover biomarkers with cardiorenal outcome and mortality as well as treatment response in patients with diabetes mellitus type 2.
Combination Therapy of RAS Inhibition and SGLT2 Inhibitors Decreases Levels of Endotrophin in Persons with Type 2 Diabetes.
Møller AL, Thöni S, Keller F, Sharifli S, Rasmussen DGK, Genovese F, Karsdal MA, Mayer G. Biomedicines. 2023 Nov 17;11(11):3084. doi: 10.3390/biomedicines11113084
DC-ren
Contact
Get involved
We are committed to collaborative R&D, and cordially invite stakeholders to open discussions eventually leading to joint initiatives.
Personalization, be it novel methods for patient stratification or adaptive design of clinical studies imposes challenges on regulatory procedures. We actively link with regulatory agencies together with experts in health technology assessment for moving personalization strategies to a next level of implementation.
You are in basic science disciplines as listed above and want to explore translation opportunities? Link with us! The DC-ren team covers a wide range of academic disciplines, including applied computer science, statistics & simulation, omics & biomarkers, all embedded in clinical research and complemented by software architecture design and implementation.
DC-ren contact
The collaborative research project DC-ren is coordinated by Univ. Prof. Dr. Gert Mayer, Medical University of Innsbruck, Austria.
Please use the following means for interacting with DC-ren:
Postal
Gert Mayer
Department of Internal Medicine IV (Nephrology and Hypertension)
Medical University Innsbruck
Anichstraße 35
6020 Innsbruck / Austria