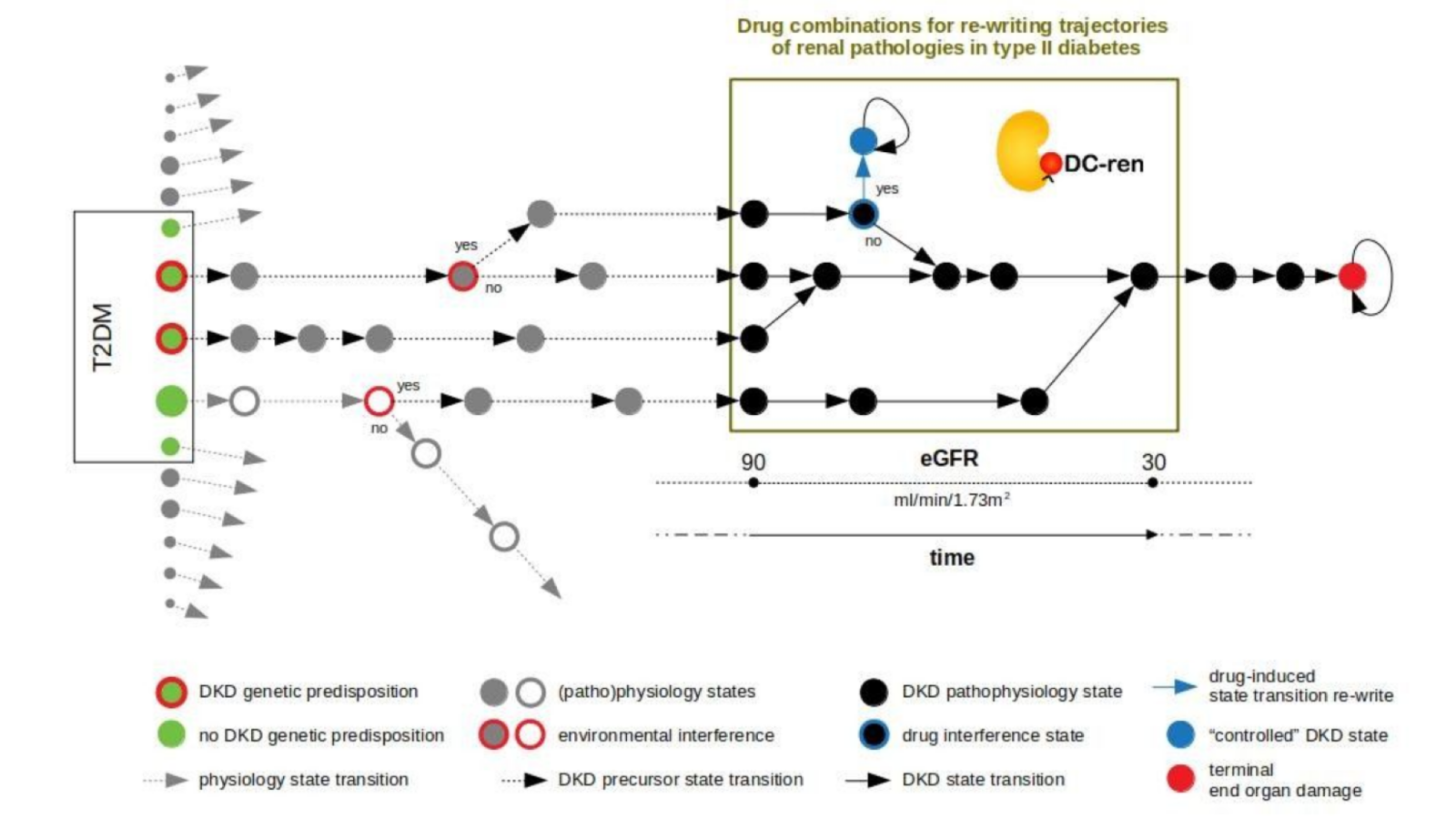
Diabetic Kidney Disease (DKD) is a serious clinical condition, and the incidence increases in parallel to the already epidemic prevalence of type 2 diabetes mellitus (T2DM). DKD is a chronic disorder, and the clinical course is currently captured by disease stages. This staging, derived from analysis of cohorts of DKD patients, allows an approximation of disease prognosis, but lacks accuracy on the level of individuals.
One purpose of assessing the prognosis is to provide guidance for treatment, and drugs for controlling various risk factors are at hand. On top, novel drugs demonstrating benefit on cardiovascular and renal outcome have been introduced to the clinics recently. However, while proving effective on a cohort level when using staging for deciding on prescription, also drug/drug combination therapy sees variance in response on the level of individuals.
Analysis of individual patients with DKD identifies inter-, but also intra-individual heterogeneity in disease evolution (prognosis) and drug response. Both, disease prognosis and the effect of a specific therapy are the direct consequence of an interaction between prevailing pathophysiology and drug mechanism of action. Hence, variability in prognosis and drug response is to be seen as variability in pathophysiology. Accordingly, personalized DKD treatment demands improved patient phenotyping for capturing this inter-individual (cross-sectional) and intra-individual (longitudinal) variability.
DC-ren follows a state-transition concept of disease evolution, a DKD state map: A combination of genetic predisposition and environmental factors leads to individualized entry paths into DKD (triggering inter-individual heterogeneity). Further disease evolution follows trajectories of changing pathophysiology states (reflected as intra-individual heterogeneity).
DC-ren aims at combining improved patient phenotyping in a state map representation of DKD: Assignment of individual patients to specific states (in contrast to more coarse-grained stages) shall offer improved estimation of prognosis. In addition, assignment to states shall inform on respective pathophysiology, in turn allowing rational selection of drugs and drug combinations promising to be effective on the level of individual patients.
The central objective of DC-ren is to transfer the state map concept into a software solution, the DC-ren toolbox, tailored at decision support for predictive statements, to be evaluated in a (virtual) clinical trial to answer the question: Shall an individual DKD patient presenting at the clinic stay on current therapy, or change to a different drug combination for stabilization of kidney function.
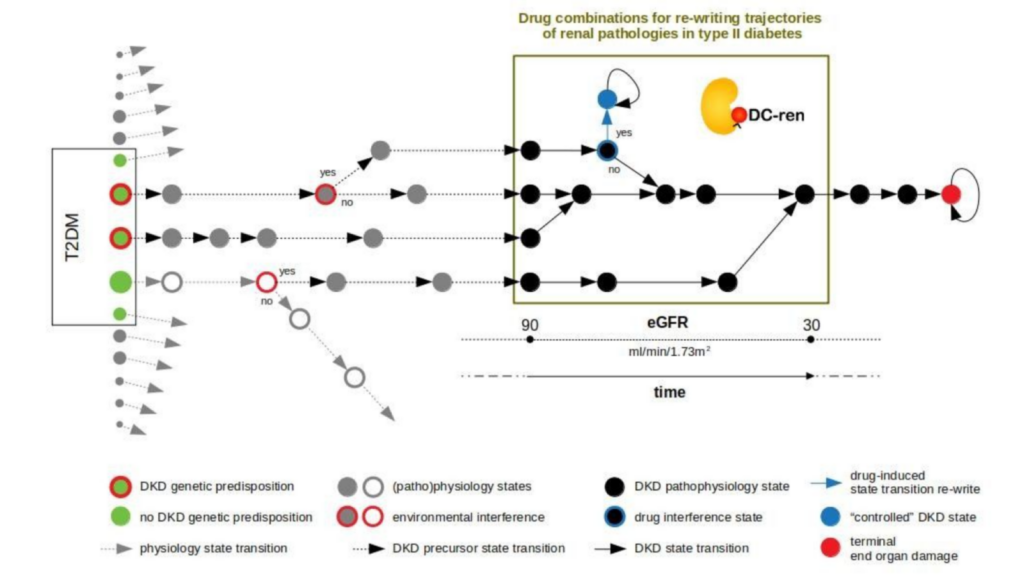
Work performed and main results achieved in the second reporting period.
Work in the first reporting period (01/2020-06/2021) focused on establishing the DC-ren data space and developing analytic methods serving the decision support logic of the DC-ren toolbox.
In the second reporting period (07/2021-12/2023) extensive sample profiling (urinary proteomics, multiplexed serum and urine protein assays and routine laboratory based expansion of clinical phenotyping) was completed. All DC-ren background and foreground data were consolidated in a central database allowing standardized data access and use by consortium members. Core analysis groups focused on the development of algorithms aiming to explain the observed variance of eGFR trajectories in the context of various therapies (blockade of the renin angiotensin system only or in combination with SGLT2 inhibitors, mineralocorticoid receptor antagonists or GLP1- agonists). Approaches were based on machine learning, dynamic systems theory, Bayesian networks and model based genetic algorithms and complemented by efforts to predict prognosis on a cohort level. The parameters finally selected for modelling and their interaction underwent clinical plausibility evaluation and appraisal of their information content with respect to pathophysiology/molecular drug mode of action. Finally, the algorithms underwent initial testing for sensitivity and specificity.
Based on work in the first reporting period DC-ren partners continued to describe aspects of the pathophysiology of DKD relevant to the response/no response to drugs of interest, which will additionally include repositioning efforts for non-steroidal anti-inflammatory agents and endothelin receptor antagonists.
Validation of these results will be a core element of the third reporting period. The protocol of a virtual clinical trial was developed that will allow to determine sensitivity and specificity of the various approaches to predict response to therapy as expressed by eGFR trajectories in independent populations. Once validated, algorithms will be part of a decision support system (a toolbox), which is a software solution that will allow physicians to enter patient data and provide an explainable output on a preferred treatment. Publication and dissemination activities informed the general and scientific public about the DC-ren activities and internal project management focused on interaction between the various involved groups.
Progress beyond the state of the art, expected results until the end of the project and potential impacts.
Cohort-centric approaches have been the gold standard for improving disease diagnosis as well as drug development, testing and prescribing. According to certain stratification means, a homogeneous cohort is defined followed by one-size-fits-all prognosis and treatment guidelines using the stratification criteria as basis. Depending on the nature of the disease such cohort approaches naturally see limits. Specifically for complex, age-associated disorders improved stratification is needed, and cross-sectional segmentation has become a widely accepted strategy. However, cross-sectional segmentation may not be sufficient. Even individuals assigned to subgroups with improved cross sectional homogeneity may still see episodes of slow and fast disease progression, and periods with excellent but also with limited response to a certain drug. This finding rests in longitudinal (intra-individual) variance caused by changing pathophysiology. Hence, an improved assessment of patients with respect to disease prognosis but also predictive statements on response to drugs needs an underlying model covering intra- and inter-individual variability of disease evolution grounding in pathophysiology. Correct stratification of patients into states demands predictive biomarkers serving as proxy for i) molecular mechanistic aspects of disease evolution and ii) informing on effect of drug mechanism of action.
State maps promise to serve the task. States are defined by proper biomarkers, the specific expression for individual patient allows state assignment. State transition information approximates a deterministic disease evolution process and covers the prognostic aspect, and prediction of drug effect indicates if a clinically detrimental state transition “re-writes” to an alternative state coming with an improved clinical situation by addition of a new drug.
DC-ren develops and exemplifies this approach for DKD, a complication seen with T2DM and concomitant significant socio-economic impact. Methods development will be integrated into a Technology Readiness Level 6 software solution (demonstration) with the promise to contribute to the wider area of personalization strategies and precision medicine.